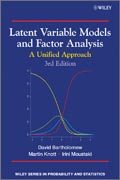
Latent variable models and factor analysis: a unified approach
Bartholomew, David J.
Knott, Martin
Moustaki, Irini
This book provides a comprehensive and unified approach to factor analysis and latent variable modeling and theory, providing a unified and coherent treatment from a statistical perspective. A general framework is presented to enablethe derivation of the commonly used models. Updated numerical examples are provided as well as the software to carry them out. Written by leading experts in the field, Latent Variable Models and Factor Analysis: Includes new topics such as, covariate effects and non-linear terms, multiple population analysis and univariate and bivariate margins. Provides a new section on structural equation models (SEM) and Markov Chain Monte Carlo methods, along with illustrative examples. Looks at estimation methods, goodness-of-fit, non-linear models, covariates, longitudinal data and multilevel modeling along with updated examples throughout. Unifies many different streams of latent variable modeling and probability modeling. An introductory section is provided, which looks at the nature and interpretation of a latent variable, motivating discussions of closely related methods which make little or no explicit use of latent variables. Principal components are discussed in more depth, exploring its relationship to factor analysis in both historical and contemporary and theoretically and empirically. Furthermore, the book explores The Bonds Model for abilities, a model which has a correlation structure which is identical to that of the factor model and hence cannot be distinguished from it and does not involve latent variables. No prior acquaintance with latent variable modeling is needed although a broad understanding of statistical theory is necessary. INDICE: Preface Acknowledgements 1 Basic Ideas and Examples 1.1 The statistical problem1.2 The basic idea 1.3 Two Examples 1.4 A broader theoretical view 1.5 Illustration of an alternative approach 1.6 An overview of special cases1.7 Principal components 1.8 The historical context 1.9 Closely related fields in Statistics 2 The General Linear Latent Variable Model 2.1 Introduction 2.2 The model 2.3 Some properties of the model 2.4 A special case 2.5 The sufficiency principle 2.6 Principal special cases 2.7 Latent variable models with non-linear terms 2.8 Fitting the models 2.9 Fitting by maximum likelihood 2.10 Fitting by Bayesian methods 2.11 Rotation2.12 Interpretation 2.13 Sampling error of parameter estimates 2.14 The prior distribution 2.15 Posterior analysis 2.16 A further note on the prior 2.17 Psychometric Inference 3 The Normal Linear Factor Model 3.1 The model 3.2 Some distributional properties 3.3 Constraints on the model 3.4 Maximum likelihood estimation 3.5 Maximum likelihood estimation by the E-M algorithm 3.6 Sampling variation of estimators 3.7 Goodness-of-fit and choice of q 3.8 Fitting without normality assumptions: Least squares methods 3.9 Other methods of fitting 3.10 Approximate methods for estimating 3.11 Goodness-of-fit and choice of q for least squares methods 3.12 Further estimation issues 3.13 Rotation and related matters 3.14 Posterior analysis: The normal case 3.15 Posterior analysis: least squares 3.16 Posterior analysis: a reliability approach 3.17 Examples 4 Binary Data: Latent Trait Models 4.1 Preliminaries 4.2 The logit/normal model4.3 The probit/normal model4.4 The equivalence of the response function and underlying variable approaches 4.5 Fitting the logit/normal model: the E-M algorithm 4.6 Sampling properties of the maximum likelihood estimators 4.7 Approximate maximum likelihood estimators 4.8 Generalised least squares methods 4.9 Goodness-of-fit 4.10 Posterior analysis 4.11Fitting the logit/normal and probit/normal models: Markov Chain Monte Carlo 4.12 Divergence of the estimation algorithm 4.13 Examples 5 Polytomous Data: Latent Trait Models 15.1 Introduction 5.2 A response function model based on thesufficiency principle 5.3 Parameter interpretation 5.4 Rotation 5.5 Maximum likelihood estimation of the polytomous logit model 5.6 An approximation to thelikelihood 5.7 Binary data as a special case 5.8 Ordering of categories 5.9 An alternative underlying variable model 5.10 Posterior analysis 5.11 Further observations 5.12 Examples of the analysis of polytomous data using the logit model 6 Latent Class Models 6.1 Introduction 6.2 The latent class model with binary manifest variables 6.3 The latent class model for binary data as a latenttrait model 6.4 Latent Classes within the GLLVM 6.5 Maximum likelihood estimation 6.6 Standard errors6.7 Posterior analysis of the latent class model with binary manifest variables 6.8 Goodness-of-Fit 6.9 Examples for binary Data6.10Latent class models with unordered polytomou
- ISBN: 978-0-470-97192-5
- Editorial: John Wiley & Sons
- Encuadernacion: Cartoné
- Páginas: 296
- Fecha Publicación: 29/07/2011
- Nº Volúmenes: 1
- Idioma: Inglés