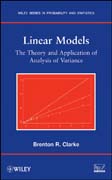
Linear models: the theory and application of analysis of variance
Clarke, Brenton R.
This graduate-level book succinctly describes the role of analysis of variance (ANOVA) in the study of linear models. Applications to linear regression (both simple and multiple) and to experimental design are included in order to help explain the theory behind and the analysis of ANOVA techniques. Throughout the book there is an equal emphasis on orthogonal representations that delve from the history of the subject and on the vector-matrix approach that has surfaced in recent years. The theory is supported with ample exercises including agenerous mix of application, theory, and real data. INDICE: Preface. Acknowledgments. 1. Introduction. 1.1 Introduction to theLinear Model and Examples. 1.2 What are the objectives?. 1.3 Problems. 2. Projection Matrices and Vector Space Theory. 2.1 Basis of a Vector Space. 2.2 Range and Kernel. 2.3 Projections. 2.3.1 Linear Model Application. 2.4 Sums and Differences of Orthogonal Projections. 2.5 Problems. 3. Least Squares Theory. 3.1 The Normal Equations. 3.2 The Gauss-Markov Theorem. 3.3 The Distribution ofS. 3.4 Some Simple Significance Tests. 3.5 Prediction Intervals. 3.6 Problems. 4. Distribution Theory. 4.1 Motivation. 4.2 Non-Central X2 and F Distributions. 4.2.1 Non-Central F-Distribution. 4.2.2 Applications to Linear Models. 4.2.3 Some Simple Extensions. 4.3 Problems. 5. Helmert Matrices and Orthogonal Relationships. 5.1 Transformations to Independent Normally Distributed Random Variables. 5.2 The Kronecker Product. 5.3 Orthogonal Components in Two-Way ANOVA: One Observation Per Cell. 5.4 Orthogonal Components in Two-Way ANOVA with Replications. 5.5 The Gauss-Markov Theorem Revisited. 5.6 Orthogonal Components for Interaction. 5.6.1 Testing for Interaction: One Observation Per Cell. 5.6.2 Example Calculation of Tukeys 1 Degree of Freedom Statistic. 5.7 Problems. 6. Further Discussion of ANOVA. 6.1 The Different Representations of OrthogonalComponents. 6.2 On the Lack of Orthogonality. 6.3 The Relationship Algebra. 6.4 The Triple Classification. 6.5 Latin Squares. 6.6 2k Factorial Designs. 6.6.1 Yates Algorithm. 6.7 The Function of Randomization. 6.8 A Brief View of Multiple Comparison Techniques. 6.9 Problems. 7. Residual Analysis: Diagnostics and Robustness. 7.1 Design Diagnostics. 7.1.1 Standardized and Studentized Residuals. 7.1.2 Combining design and residual effects on fit - DFITS. 7.1.3 The Cook-D-Statistic. 7.2 Robust Approaches. 7.2.1 Adaptive Trimmed Likelihood Algorithm. 7.3 Problems. 8. Models Including Variance Components. 8.1 The One-Way Random Effects Model. 8.2 The Mixed Two-Way Model. 8.3 A Split Plot Design. 8.3.1 A Traditional Model. 8.4 Problems. 9. Likelihood Approaches. 9.1 Maximum Likelihood Estimation. 9.2 REML. 9.3 Discussion of Hierarchical Statistical Models. 9.3.1 Hierarchy for the Mixed Model (Assuming Normality). 9.4 Problems. 10. Uncorrelated Residuals Formed from the Linear Model. 10.1 Best Linear Unbiased Error Estimates. 10.2 The Best Linear Unbiased Scalar-Covariance-Matrix Approach. 10.3 An Explicit Solution. 10.4 The Recursive Residuals. 10.4.1 The Recursive Residuals and their Properties. 10.5 Uncorrelated Residuals. 10.5.1 The Main Results. 10.5.2 Final Remarks. 10.6 Problems. 11. Further inferential questions relating to ANOVA. 12. Permissions. Glossary. Bibliography. References. Topic Index.
- ISBN: 978-0-470-02566-6
- Editorial: John Wiley & Sons
- Encuadernacion: Cartoné
- Páginas: 254
- Fecha Publicación: 01/08/2008
- Nº Volúmenes: 1
- Idioma: Inglés