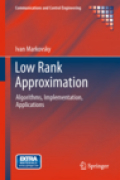
Data Approximation by Low-complexity Models details the theory, algorithms, and applications of structured low-rank approximation. Efficient local optimization methods and effective suboptimal convex relaxations for Toeplitz, Hankel,and Sylvester structured problems are presented. Much of the text is devoted to describing the applications of the theory including: system and control theory; signal processing; computer algebra for approximate factorization and common divisor computation; computer vision for image deblurring and segmentation; machine learning for information retrieval and clustering; bioinformatics for microarray data analysis; chemometrics for multivariate calibration; and psychometrics for factor analysis. Software implementation of the methods is given, making the theory directly applicable in practice. All numerical examples are included in demonstration files giving hands-on experience and exercises and MATLAB® examples assist in the assimilation of the theory. Provides the reader with an analysis tool which is more generally applicable than the commonly-used total least squares. Shows the reader solutions to the problem of data modelling by linear systems from a sweeping field of applications. Supplementary electronic and class-based materials will aid tutors in presenting this material to their students. INDICE: Introduction. From Data to Models. Applications in System and Control Theory. Applications in Signal Processing. Applications in Computer Algebra. Applications in Machine Learing. Subspace-type Algorithms. Algorithms Basedon Local Optimization. Data Smoothing and Filtering. Recursive Algorithms.
- ISBN: 978-1-4471-2226-5
- Editorial: Springer London
- Encuadernacion: Cartoné
- Páginas: 254
- Fecha Publicación: 30/11/2011
- Nº Volúmenes: 1
- Idioma: Inglés