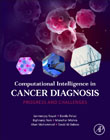
Computational Intelligence in Cancer Diagnosis: Progress and Challenges
Nayak, Dr. Janmenjoy
Pelusi, Danilo
Naik, Bighnaraj
Mishra, Manohar
Muhammad, Khan
Al-Dabass, David
Computational Intelligence in Cancer Diagnosis: Progress and Challenges provides insights into the current strength and weaknesses of different applications and research findings on computational intelligence in cancer research. The book improves the exchange of ideas and coherence among various computational intelligence methods and enhances the relevance and exploitation of application areas for both experienced and novice end-users. Topics discussed include neural networks, fuzzy logic, connectionist systems, genetic algorithms, evolutionary computation, cellular automata, self-organizing systems, soft computing, fuzzy systems, and hybrid intelligent systems. The book's chapters are written by international experts from both cancer research, oncology and computational sides to cover different aspects and make it comprehensible for readers with no background on informatics. Contains updated information about advanced computational intelligence, spanning the areas of neural networks, fuzzy logic, connectionist systems, genetic algorithms, evolutionary computation, cellular automata, self-organizing systems, soft computing, fuzzy systems, and hybrid intelligent systems in diagnosing cancer diseases Discusses several cancer types, including their detection, treatment and prevention Presents case studies that illustrate the applications of intelligent computing in data analysis to help readers to analyze and advance their research in cancer INDICE: SECTION 1. Introduction to Computational Intelligence Approaches 1. The Roadmap to the Adoption of Computational Intelligence in Cancer Diagnosis: the Clinical-Radiological Perspective 2. Deep Learning Approaches for High Dimensional Cancer Microarray Data Feature Prediction: A Review 3. Integrative Data Analysis and Automated Deep Learning Technique for Ovary Cancer Detection 4. Learning from Multiple Modalities of Imaging Data for Cancer diagnosis 5. Neural Network for Lung Cancer Diagnosis 6. Machine Learning for Thyroid Cancer Diagnosis SECTION 2. Prediction of Cancer Susceptibility 7. Machine Learning based Detection and Classification of Lung Cancer 8. Deep learning techniques for Oral Cancer Diagnosis 9. An Intelligent Deep Learning approach for Colon cancer diagnosis 10. Effect of COVID-19 on cancer patients: Issues and Future Challenges 11. Empirical Wavelet Transform-based Fast Deep Convolutional Neural Network for Detection and Classification of Melanoma SECTION 3. Advance Computational Intelligence Paradigms 12. Convolutional neural networks and stacked generalization ensemble method in breast cancer prognosis 13. An Advance Ensemble Learning model for Cancer diagnosis 14. Deep Learning Based Computer-Aided Cervical Cancer Diagnosis in Digital histopathology Images 15. Deep Learning Techniques for Hepatocellular Carcinoma Diagnosis 16. Issues and Future Challenges in Cancer prognosis: (Prostate cancer: A case study) 17. A Novel Cancer Drug Target Module Mining Approach using Non-Swarm Intelligence
- ISBN: 978-0-323-85240-1
- Editorial: Academic Press
- Encuadernacion: Rústica
- Páginas: 600
- Fecha Publicación: 01/06/2022
- Nº Volúmenes: 1
- Idioma: Inglés