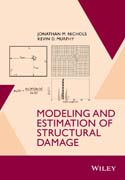
Modeling and Estimation of Structural Damage
Nichols, Jonathan M.
Murphy, Kevin D.
Modelling and Estimation of Damage in Structures is a comprehensiveguide to solving the type of modelling and estimation problems associated with the physics of structural damage. Provides a model–based approach to damage identification Presents an in–depth treatment of probability theory and random processes Covers both theory and algorithms for implementing maximum likelihood and Bayesian estimation approaches Includes experimental examples of all detection and identification approaches Provides a clear means by which acquired data can be used to make decisions regarding maintenance and usage of a structure INDICE: Preface ix .1 Introduction 1 .1.1 Users Guide 1 .1.2 Modeling & Estimation Overview 2 .1.3 Motivation 4 .1.4 Structural Health Monitoring 7 .1.4.1 Data–driven Approaches 10 .1.4.2 Physics Based Approach 14 .1.5 Organization & Scope 16 .2 Probability 21 .2.1 Probability Basics 23 .2.2 Probability Distributions 25 .2.3 Multivariate Distributions, Conditional Probability, and Independence 28 .2.4 Functions of Random Variables 31 .2.5 Expectations and Moments 38 .2.6 Moment Generating Functions & Cumulants 42 .3 Random Processes 51 .3.1 Properties of a Random Process 54 .3.2 Stationarity 58 .3.3 Spectral Analysis 61 .3.3.1 Spectral Representation of Deterministic Signals 62 .3.3.2 Spectral Representation of Stochastic Signals 66 .3.3.3 Power Spectral Density 68 .3.3.4 Relationship to Correlation Functions 72 .3.3.5 Higher–order Spectra 75 .3.4 Markov Models 83 .3.5 Information Theoretics 83 .3.5.1 Mutual Information 87 .3.5.2 Transfer Entropy 89 .3.6 Random Process Models for Structural Response Data 93 .4 Modeling in Structural Dynamics 99 .4.1 Why Build Mathematical Models? 100 .4.2 Good Versus Bad Models – An Example 101 .4.3 Elements of Modeling 104 .4.3.1 Newton s Laws 104 .4.3.2 Background to Variational Methods 105 .4.3.3 Variational Mechanics 107 .4.3.4 Lagrange s Equations 108 .4.3.5 Hamilton s Principle 112 .4.4 Common Challenges 117 .4.4.1 Impact Problems 117 .4.4.2 Stress Singularities & Cracking 120 .4.5 Solution Techniques 122 .4.5.1 Analytical Techniques I – Ordinary Differential Equations 123 .4.5.2 Analytical Techniques II – Partial Differential Equations 132 .4.5.3 Local Discretizations 135 .4.5.4 Global Discretizations 136 .4.6 Volterra Series for Nonlinear Systems 137 .5 Physics Based Model Examples 147 .5.1 Imperfection Modeling in Plates 147 .5.1.1 Cracks as Imperfections 147 .5.1.2 Boundary Imperfections: In–Plane Slippage 148 .5.2 Delamination in a Composite Beam 155 .5.3 Bolted Joint Degradation: Quasi–static Approach 164 .5.3.1 The Model 164 .5.3.2 Experimental System and Procedure 168 .5.3.3 Results and Discussion 169 .5.4 Bolted Joint Degradation: Dynamic Approach 172 .5.5 Corrosion Damage 181 .5.6 Beam on a Tensionless Foundation 185 .5.6.1 Equilibrium Equations and Their Solution 187 .5.6.2 Boundary Conditions 188 .5.6.3 Results 189 .5.7 Cracked, Axially Moving Wires 192 .5.7.1 Some Useful Concepts from Fracture Mechanics 192 .5.7.2 The Effect of a Crack on the Local Stiffness 194 .5.7.3 Limitations 196 .5.7.4 Equations of Motion 198 .5.7.5 Natural Frequencies and Stability 199 .5.7.6 Results 200 .6 Estimating Statistical Properties of Structural Response Data 205 .6.1 Estimator Bias and Variance 208 .6.2 Method of Maximum Likelihood 211 .6.3 Ergodicity 215 .6.4 Power Spectral Density and Correlation Functions for LTI Systems 220 .6.4.1 Estimation of Power Spectral Density 221 .6.4.2 Estimation of Correlation Functions 238 .6.5 Estimating Higher Order Spectra 245 .6.5.1 Coherence Functions 251 .6.5.2 Bispectral Density Estimation 252 .6.5.3 Anaytical Bicoherence for Non–Gaussian Signals 259 .6.5.4 Trispectral Density Function 267 .6.6 Estimation of Information Theoretics 278 .6.7 Generating Random Processes 287 .6.7.1 Review of Basic Concepts 288 .6.7.2 Data with a Known Covariance and Gaussian Marginal PDF 290 .6.7.3 Data with a Known Covariance and Arbitrary Marginal PDF 294 .6.7.4 Examples 299 .6.8 Stationarity Testing 305 .6.8.1 Reverse Arrangement Test 305 .6.8.2 Evolutionary Spectral Testing 308 .6.9 Hypothesis Testing & Intervals of Confidence 314 .6.9.1 Detection Strategies 314 .6.9.2 Detector Performance 321 .6.9.3 Intervals of Confidence 329 .7 Parameter Estimation for Structural Systems 337 .7.1 Method of Maximum Likelihood 340 .7.1.1 Linear Least Squares 343 .7.1.2 Finite Element Model Updating 345 .7.1.3 Modified Differential Evolution for Obtaining MLEs 348 .7.1.4 Structural Damage MLE Example 353 .7.1.5 Estimating Time–of–Flight for Ultrasonic Applications 356 .7.2 Bayesian Estimation 368 .7.2.1 Conjugacy 370 .7.2.2 Using Conjugacy to Assess Algorithm Performance 371 .7.2.3 Markov Chain Monte Carlo (MCMC) Methods 379 .7.2.4 Gibbs Sampling 384 .7.2.5 Conditional Conjugacy: Sampling the Noise Variance 385 .7.2.6 Beam Example Revisited 388 .7.2.7 Population–based MCMC 390 .7.3 Multi–model Inference 395 .7.3.1 Model Comparison via AIC 396 .7.3.2 Reversible Jump MCMC 401 .8 Detecting Damage–induced Nonlinearity 409 .8.1 Capturing Nonlinearity 413 .8.1.1 Higher–order cumulants 414 .8.1.2 Higher–order Spectral Coefficients 416 .8.1.3 Nonlinear Prediction Error 418 .8.1.4 Information Theoretics 420 .8.2 Bolted Joint Revisited 422 .8.2.1 Composite Joint Experiment 422 .8.2.2 Kurtosis results 424 .8.2.3 Spectral results 427 .8.3 Bispectral Detection: the SDOF, Gaussian Case 429 .8.3.1 Bispectral Detection Statistic 429 .8.3.2 Test Statistic Distribution 430 .8.3.3 Detector Performance 432 .8.4 Bispectral Detection: the General MDOF Case 437 .8.4.1 Bicoherence Detection Statistic Distribution 440 .8.4.2 Which Bicoherence to Compute? 441 .8.4.3 Optimal Input Probability Distribution for Detection 444 .8.5 Application of the HOS to Delamination Detection 446 .8.6 Method of Surrogate Data 454 .8.6.1 Fourier Transform–Based Surrogates 456 .8.6.2 AAFT Surrogates 457 .8.6.3 IAFFT Surrogates 458 .8.6.4 DFT Surrogates 459 .8.7 Numerical Surrogate Examples 460 .8.7.1 Detection of Bi–linear Stiffness 460 .8.7.2 Detecting Cubic Stiffness 466 .8.7.3 Surrogate Invariance to Ambient Variation 469 .8.8 Surrogate Experiments 472 .8.8.1 Detection of Rotor–Stator Rub 472 .8.8.2 Bolted Joint Degradation with Ocean Wave Excitation 474 .8.9 Surrogates for Non–stationary Data 482 .8.10 Chapter Summary 483 .9 Damage Identification 489 .9.1 Modeling & Identification of Imperfections in Shell Structures 489 .9.1.1 Modeling of Submerged Shell Structures 490 .9.1.2 Non–contact Results Using Maximum Likelihood 494 .9.1.3 Bayesian Identification of Dents 500 .9.1.4 Displacement as the Observable 508 .9.2 Modeling & Identification of Delamination 512 .9.3 Modeling & Identification of Cracked Structures 519 .9.3.1 Cracked Plate Model 519 .9.3.2 Crack Parameter Identification 521 .9.3.3 Optimization of Sensor Placement 533 .9.4 Modeling & Identification of Corrosion 537 .9.4.1 Experimental Setup 541 .9.4.2 Results and Discussion 542 .9.5 Chapter Summary 547 .10 Decision Making in Condition–based Maintenance 555 .10.1 Structured Decision Making 556 .10.2 Example: Ship in Transit 557 .10.2.1 Loading Data 559 .10.2.2 Ship Stringer Model 564 .10.2.3 Cumulative Fatigue Model 571 .10.3 Optimal Transit 574 .10.3.1 Problem Statement 574 .10.3.2 Solutions via Dynamic Programming 575 .10.3.3 Transit Examples 577 .10.4 Summary 581 .A Useful Constants and Probability Distributions 585 .B Contour Integration of Spectral Density Functions 589 .C Derivation of Terms for the Trispectrum of an M–DOF Nonlinear Structure 595
- ISBN: 978-1-118-77705-3
- Editorial: Wiley–Blackwell
- Encuadernacion: Cartoné
- Páginas: 450
- Fecha Publicación: 05/02/2016
- Nº Volúmenes: 1
- Idioma: Inglés