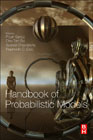
Handbook of Probabilistic Models
Samui, Pijush
Tien Bui, Dieu
Chakraborty, Subrata
Deo, Ravinesh
Used to define the risk in any engineering project, the development and the appropriate application of probabilistic models is a critical part of the design and analysis process in any engineering operation. A definitive reference for understanding and creating more effective models, Handbook of Probabilistic Models for Researchers, Engineers and Scientist carefully examines the applications of advanced probabilistic models in conventional engineering fields. In this Handbook, practitioners, researchers, and scientist will find detailed explanations of the technical concept, applications of the proposed methods and the respective scientific approaches to solve the problem. Handbook of Probabilistic Models for Researchers, Engineers and Scientist provides an interdisciplinary approach to create advanced probabilistic models for engineering fields ranging from conventional fields of mechanical engineering, civil engineering to electronics, electrical, earth sciences, climate, agriculture, water resource, mathematical sciences and computer sciences. The authors cover topics such as: minimax probability machine regression, stochastic finite element method, relevance vector machine, logistic regression, Monte Carlo simulations, random matrix, Gaussian process regression, kalman filter, stochastic optimization, maximum likelihood, bayesian inference, bayesian update, kriging, copula-statistical models, etc. Explains the application of advanced probabilistic models encompassing multi-disciplinary research Application of probabilistic modelling to various emerging areas in Engineering Provides and interdisciplinary approach to probabilistic models and their applications to solve a wide range of practical problems. INDICE: 1. Monte Carlo Simulation2. Stochastic Optimization Method3. Reliability Analysis4. Stochastic Finite Element Method5. Kalman Filter6. Random matrix7. Markov Chain8. Gaussian Process Regression9. Logistic regression10. Geostatistics11. Kriging12. Bayesian inference13. Bayesian updating14. Probabilistic Neural Network15. SVM, Relevance vector machine
- ISBN: 978-0-12-816514-0
- Editorial: Butterworth-Heinemann
- Encuadernacion: Rústica
- Páginas: 612
- Fecha Publicación: 01/09/2019
- Nº Volúmenes: 1
- Idioma: Inglés