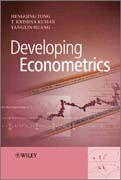
Developing econometrics: statistical theories and methods with applications to economics and business
Tong, Hengqing
Kumar, T. Krishna
Huang, Yangxin
Statistical Theories and Methods with Applications to Economics and Business highlights recent advances in statistical theory and methods that benefit econometric practice. It deals with exploratory data analysis, a prerequisite to statistical modelling and part of data mining. It provides recently developed computational tools useful for data mining, analysing the reasons to do data mining and the best techniques to use in a given situation. Provides a detailed description of computer algorithms. Provides recently developed computational tools useful for data mining Highlights recent advances in statistical theory and methods that benefit econometric practice. Features examples with real life data. Accompanying software featuring DASC (Data Analysis and Statistical Computing). Essential reading for practitioners in any area of econometrics; business analysts involved in economics and management; and Graduate students andresearchers in economics and statistics. ÍNDICE: Foreword. Preface. Chapter 1 Introduction. 1.1 Nature and Scope ofEconometrics. 1.2 Types of Economic Problems, Types of Data, and Types of Models. 1.3 Pattern Recognition and Exploratory Data Analysis. 1.4 Econometric Modelling: The Roadmap of This Book. Chapter 2 Independent Variables in Linear Regression Models. 21 Brief Review of Linear Regression. 2.2 Selection of Independent Variable and Stepwise Regression. 2.3 Multivariate Data Transformation and Polynomial Regression. 2.4 Column Multicollinearity in Design Matrix and Ridge Regression. 2.5 Recombination of Independent Variable and Principal Components Regression. Chapter 3 Alternate Structures of Residual Error in Linear Regression Models. 31 Heteroscedasticity : Consequences and Tests for its Existence. 3.2 Generalized Linear Model with Covariance Being a Diagonal Matrix. 3.3 Autocorrelation in a Linear Model. 3.4 Generalized Linear Model with Positive Definite Covariance Matrix. 35 Random Effect and Variance Component Model. Chapter 4 Discrete Variables and Nonlinear Regression Models. 4.1 Regression Model When Independent Variables Are Categorical. 4.2 Models with Categorical orDiscrete Dependent Variables. 4.3 Nonlinear Regression Model and Its Algorithm. 4.4 Nonlinear Regression Models in Practice. Chapter 5 Nonparametric and Semiparametric Regression Models. 5.1 Nonparametric Regression and Weight Function Method. 5.2 Semiparametric Regression Model. 5.3 Stochastic Frontier Regression Model. Chapter 6 Simultaneous Equations Model and Distributed Lag Models.6.1 Simultaneous Equations Models and Inconsistency of OLS Estimators. 6.2 Statistical Inference for Simultaneous Equations Model. 6.3 The Concepts of Lag Regression Models. 6.4 Finite Distributed Lag Models. 6.5 Infinite DistributedLag Models. Chapter 7 Stationary Time Series Models. 7.1 Autoregression ModelAR(p). 7.2 Moving Average Model MA(q). 7.3 Auto-Regressive Moving-Average Process ARMA(p,q). Chapter 8 Nonstationary and Multivariate Time Series Models. 8.1 Multivariate Stationary Time Series Model. 8.2 Nonstationary Time Series and Unit Root Process. 8.3 Cointegration and Error Correction. 8.4 Autoregression Conditional Heteroscedasticity Model in Time Series. 8.5 Mixed Models of Multivariate Regression with Time. Chapter 9 Multivariate Statistical Analysis and Data Analysis. 9.1 Model of Analysis of Variance. 9.2 Other Multivariate Statistical Analysis Models. 9.3 Customer Satisfaction Model and Path Analysis. 9.4 Data Analysis and Process. Chapter 10 Summary and Further Discussions. 10.1About Probability Distributions: Parametric and Non-parametric. 10.2 Regression. 10.3 Model Specification and Prior Information. 10.4 Classical Theory of Statistical Inference. 10.5 Computation of Maximum Likelihood Estimates. 10.6 Specification Searche. 10.7 Resampling and Sampling Distributions-The Bootstraps Method. 10.8 Bayesian Inference. Index.
- ISBN: 978-0-470-68177-0
- Editorial: John Wiley & Sons
- Encuadernacion: Cartoné
- Páginas: 480
- Fecha Publicación: 18/11/2011
- Nº Volúmenes: 1
- Idioma: Inglés